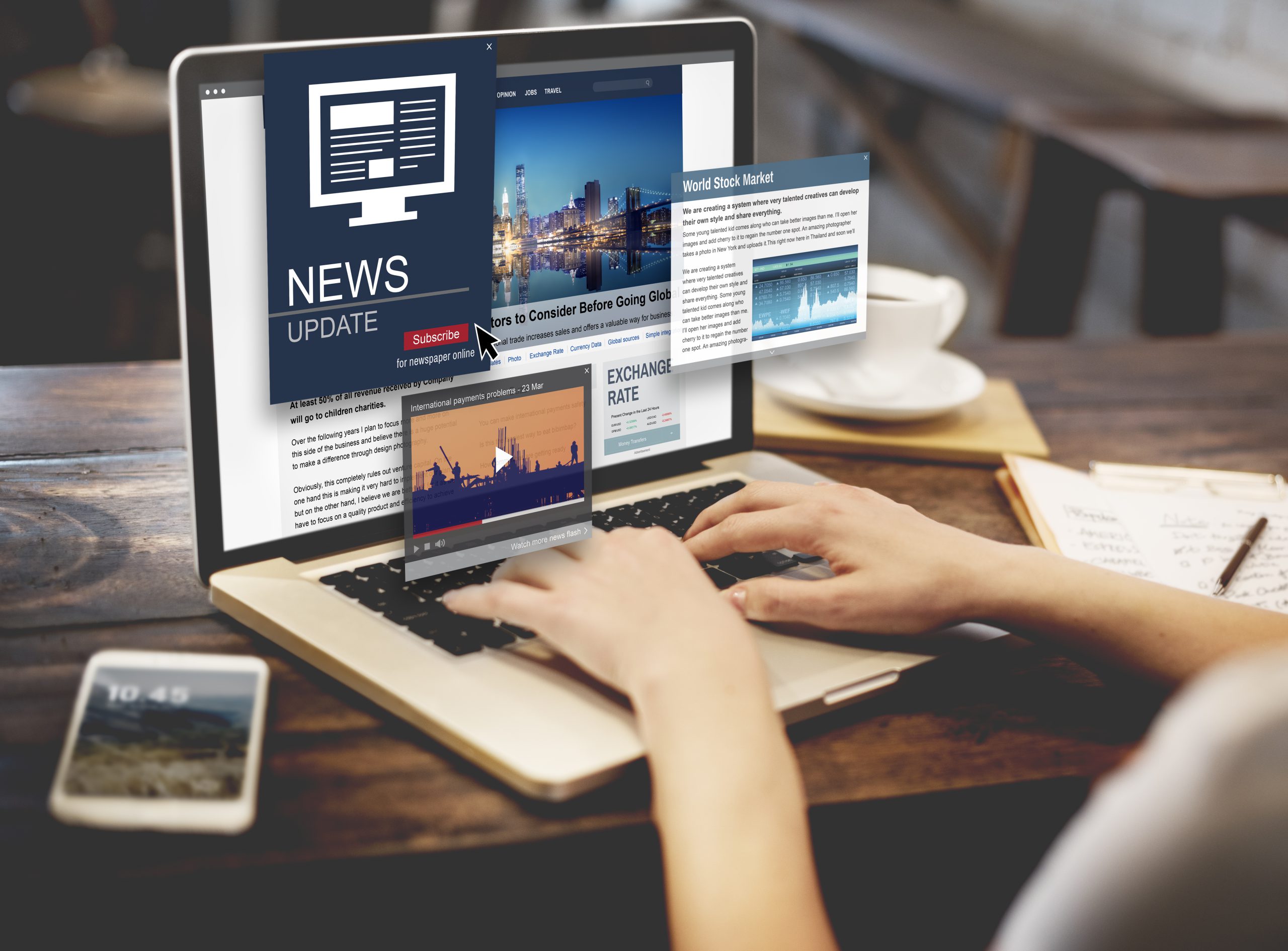
News
What’s happening with the Colive Voice Project?
Stay in the know with what’s happening with our digital health study
-
10 May 2023
Developing vocal biomarker research
-
25 Apr 2023
Digital Health Study: Colive Voice Team Interview
-
25 Apr 2023
Easing the screening of diabetes through the voice
-
25 Oct 2022
How to detect diseases and monitor our health easily using voice?
-
03 Jun 2022
Women raise their voices to advance cancer research
-
19 May 2022
A voice to help shape the future of healthcare
-
13 May 2022
Colive Voice project sprints forward
-
06 Mar 2022
Colive Voice researchers will share the first results on diabetes at SFD conference 2022
-
05 Dec 2021
Colive Voice is looking for participants with chronic diseases
-
29 Nov 2021
Guy Fagherazzi, participates in the SciLux podcast